TOP
Research
Research Teams
High Performance Big Data Research Team
High Performance Big Data Research Team
Japanese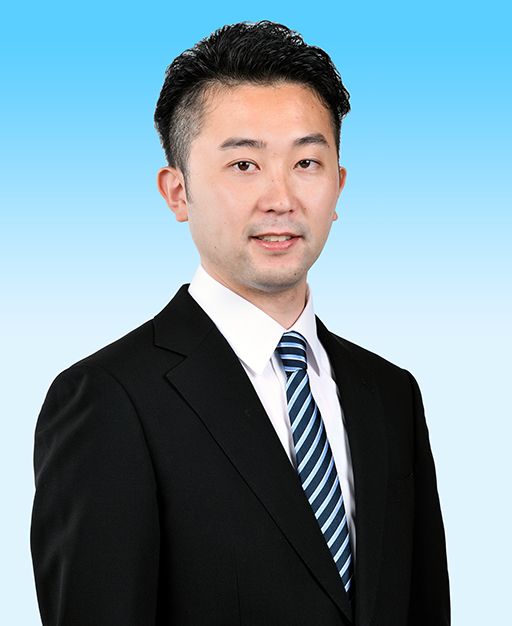
Team Leader Kento Sato
kento.sato[at]riken.jp (Lab location: Kobe)
- Please change [at] to @
- 2024
- Unit Leader, Data Management Platform Development Unit, AI for Science Platform Division, R-CCS, RIKEN (-present)
- 2018
- Team leader, High Performance Big Data Research Team, R-CCS, RIKEN (-present)
- 2018
- Invited Senior Researcher, RWBC-OIL, National Institute of Advanced Industrial Science and Technology (AIST), Japan.
- 2017
- Invited Senior Researcher, RWBC-OIL, National Institute of Advanced Industrial Science and Technology (AIST), Japan.
- 2017
- Computer Scientist, Center for Applied Scientific Computing (CASC), Lawrence Livermore National Laboratory (LLNL), USA.
- 2014
- Postdoctoral Researcher, Center for Applied Scientific Computing (CASC), Lawrence Livermore National Laboratory (LLNL), USA.
- 2014
- Postdoctoral Researcher, Global Scientific Information and Computing Center (GSIC), Tokyo Institute of Technology, Japan
- 2014
- Ph.D. in Science, Department of Mathematical and Computing, Tokyo Institute of Technology, Japan.
Keyword
- Big Data Processing Platform
- Machine Learning/Deep Learning Platform
- Fault Tolerance
- File system
- Virtualization and container technologies
Research summary
The High-Performance Big Data Research Team at the RIKEN Center for Computational Science has be developing system software for the advancement of high-performance computers such as the Fugaku supercomputer. In particular, we aim to integrate high performance computing (HPC), big data (Big Data), and artificial intelligence (AI). To achieve this goal, we are researching and developing fundamental technologies that are universally required for the advancement of high-performance computing. Especially, we develop system software for accelerating big data processing and AI training & inference (i.e., HPC for Big Data/AI) while we also make use of big data and AI technologies for the advancement of high-performance computing (i.e., Big Data/AI for HPC). We also study technologies for designing future high-performance computers. Specifically, we are developing technologies for scalable parallel I/O, scalable machine learning and deep learning using hierarchical memory storage technology, utilizing non-volatile memory, scalable and efficient fault-tolerant technology, efficient data compression and transfer on high-speed networks, and advanced programming environments. We also explore architectures to develop the next generation of large-scale systems. We are actively collaborating with researchers from domestic and foreign companies, universities, and national laboratories to establish a high-performance big data processing infrastructure.
Main research results
Compression of Time Evolutionary Image Data through Predictive Deep Neural Networks
Recent advances in deep neural networks (DNNs) have made it easier to predict the time evolutionary data. In this study, we have developed a compression framework (TEZIP) that utilizes AI technology. In TEZIP, a Recurrent Neural Network called PredNet is used to learn the temporal evolution of the target data. As a result of TEZIP's evaluation, TEZIP achieves up to 3.2 times higher compression ratio than existing lossless compression methods such as x265, and up to 3.3 times than lossless compression methods such as SZ.
Representative papers
-
Taiyu Wang, Qinglin Yang, Kaiming Zhu, Junbo Wang, Chunhua Su, Kento Sato,
“LDS-FL: Loss Differential Strategy based Federated Learning for Privacy Preserving,”
in IEEE Transactions on Information Forensics and Security, doi: 10.1109/TIFS.2023.3322328. , 2023 -
Takaaki Fukai, Kento Sato and Takahiro Hirofuchi,
“Analyzing I/O Performance of a Hierarchical HPC Storage System for Distributed Deep Learning”,
The 23rd International Conference on Parallel and Distributed Computing, Applications and Technologies (PDCAT’22), December, 2022, Sendai, Japan -
Xi Zhu, Junbo Wang, Wuhui Chen, Kento Sato,
“Model compression and privacy preserving framework for federated learning”,
Future Generation Computer Systems, 2022, ISSN 0167-739X, https://doi.org/10.1016/j.future.2022.10.026 -
Amitangshu Pal, Junbo Wang, Yilang Wu, Krishna Kant, Zhi Liu, Kento Sato,
“Social Media Driven Big Data Analysis for Disaster Situation Awareness: A Tutorial”,
in IEEE Transactions on Big Data, doi: 10.1109/TBDATA.2022.3158431, Mar., 2022 -
Feiyuan Liang, Qinglin Yang, Ruiqi Liu, Junbo Wang, Kento Sato, Jian Guo,
“Semi-Synchronous Federated Learning Protocol with Dynamic Aggregation in Internet of Vehicles,”
in IEEE Transactions on Vehicular Technology, doi: 10.1109/TVT.2022.3148872, Feb., 2022 -
Akihiro Tabuchi, Koichi Shirahata, Masafumi Yamazaki, Akihiko Kasagi, Takumi Honda, Kouji Kurihara, Kentaro Kawakami, Tsuguchika Tabaru, Naoto Fukumoto, Akiyoshi Kuroda, Takaaki Fukai and Kento Sato,
“The 16,384-node Parallelism of 3D-CNN Training on An Arm CPU based Supercomputer”,
28th IEEE International Conference on High Performance Computing, Data, and Analytics (HiPC2021), Nov, 2021 -
Steven Farrell, Murali Emani, Jacob Balma, Lukas Drescher, Aleksandr Drozd, Andreas Fink, Geoffrey Fox, David Kanter, Thorsten Kurth, Peter Mattson, Dawei Mu, Amit Ruhela, Kento Sato,,Koichi Shirahata, Tsuguchika Tabaru, Aristeidis Tsaris, Jan Balewski, Ben Cumming, Takumi Danjo, Jens Domke, Takaaki Fukai, Naoto Fukumoto, Tatsuya Fukushi, Balazs Gerofi, Takumi Honda, Toshiyuki Imamura, Akihiko Kasagi, Kentaro Kawakami, Shuhei Kudo, Akiyoshi Kuroda, Maxime Martinasso, Satoshi Matsuoka, Kazuki Minami, Prabhat Ram, Takashi Sawada, Mallikarjun Shankar, Tom St. John, Akihiro Tabuchi, Venkatram Vishwanath, Mohamed Wahib, Masafumi Yamazaki, Junqi Yin and Henrique Mendonca,
“MLPerf HPC: A Holistic Benchmark Suite for Scientific Machine Learning on HPC Systems”,
The Workshop on Machine Learning in High Performance Computing Environments (MLHPC) 2021 in conjunction with SC21, Nov, 2021 -
Rupak Roy, Kento Sato, Subhadeep Bhattacharya, Xingang Fang, Yasumasa Joti, Takaki Hatsui, Toshiyuki Hiraki, Jian Guo and Weikuan Yu:
“Compression of Time Evolutionary Image Data through Predictive Deep Neural Networks”
In proceedings of the 21 IEEE/ACM International Symposium on Cluster, Cloud and Internet Computing (CCGrid 2021), (2021). -
Tonmoy Dey, Kento Sato, Bogdan Nicolae, Jian Guo, Jens Domke, Weikuan Yu, Franck Cappello, and Kathryn Mohror:
“Optimizing Asynchronous Multi-Level Checkpoint/Restart Configurations with Machine Learning”
The IEEE International Workshop on High-Performance Storage, (2020). -
Chapp, D., Rorabaugh, D., Sato, K., Ahn, D. H., & Taufer, M:
“A three-phase workflow for general and expressive representations of nondeterminism in HPC applications”
The International Journal of High Performance Computing Applications, 33(6), 1175–1184. (2019).